COTAN project
COTAN is a mathematical model and a statistical framework to study an important category of biological datasets.
In recent years there was an exceptional growth of omics techniques and approaches. One of the most important is transcriptomics, that is the detection of RNA molecules inside the cells, also called RNA-seq, of which there are several kinds. The oldest is bulk RNA-seq, that can only count RNA molecules in sets of thousands of cells. Its evolution, works at the level of single cells and hence is called scRNA-seq.
Single-cell RNA sequencing comes with several drawbacks. The first is its bad SNR, as it is based on PCR amplification (an exponential process which is more apt to qualitative detection of few genes, than quantitative genome-wide analysis). The second is its very low efficiency, that leads to dropout artefacts (random disappearence of genes that should be present).
While not much can be done to solve the latter, the recent introduction of unique molecular identifiers (UMI) almost solved the former, allowing several authors to deal with the low efficiency through suitable probability models. COTAN is our attempt in this direction and it is described in [15].
Excerpt from the introduction: COTAN is based on three main elements: a robust estimation of the UMI detection efficiency (UDE) of each cell, a flexible model for the probability of zero UMI counts, and a generalized contingency table framework for zero/non-zero UMI counts for couples of genes. In fact, COTAN estimates the co-expression of gene pairs by comparing the number of cells that have zero UMI counts for both genes, with the expected value under independence hypothesis. This approach is based on the fact that technical zeros are always independently distributed, while biological zeros may be correlated for genes associated to cell differentiation, providing a way to recover information on the joint distribution.
Our model assumes that for each gene there is a real expression Λ with unknown distribution, and that every cell has a coefficient ν for the detection efficiency there. The actual read count is modelled as a discrete random variable R with conditional Poisson distribution with mean νΛ.
We use the method of moments to estimate the parameters, and then compute the estimated probability that a gene-cell results in R=0 molecule counts. To this end, we include another parameter a for each gene, and fit a simple universal family of functions for this probability.
Then we can compute classical 2x2 contingency tables for all couples of genes and use the expected probability of R=0 to get the "expected" numbers under the independence hypothesis.
The inference is then performed as usual with 1 df chi-squared distribution, as was verified with simulated data.
One of the main outputs of the analysis is a matrix of correlation of genes, which proved to be much more robust than Pearson's or Spearman's with this kind of data.
[15] | COTAN: scRNA-seq data analysis based on gene co-expression | Galfrè, Morandin, Pietrosanto, Cremisi, Helmer-Citterich | NAR Genomics | 2021 |
![]() |
![]() |
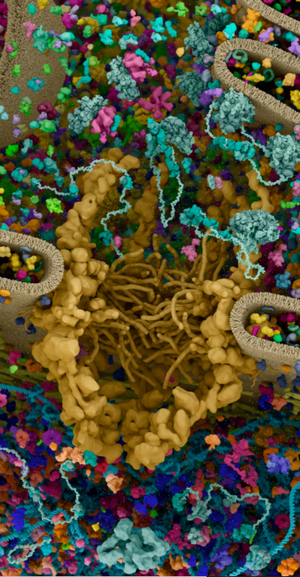